Not all patients are suitable candidates for HIFU treatment, which currently limits the treatment efficacy and success rate of the therapy. Clinical criteria have been formed for the pre-treatment patient selection, but these are usually only MRI-based and lack more comprehensive inclusion of medical aspects such as patient anatomy and treatment history. Furthermore, the complex nonlinear relationships between these parameters the treatment outcome are often not taken into account when regression analysis is conducted. Therefore, more sophisticated models are needed in order to evaluate the suitability of patients for HIFU treatments and predicting the treatment outcome.
Machine learning models have recently gained popularity in clinical decision making due to their ability to incorporate multiple parameters into a model and find underlying nonlinear relationships between these parameters and the final outcome. Different machine learning models, such as neural networks, can be trained using patient data from the treatment in order to empirically learn these relationships from a dataset containing the representative cases. Given these benefits, machine learning models have great potential in predicting the outcome of nontrivial medical cases.
A diagram illustrating a simple neural network model for HIFU treatment outcome prediction is shown in the figure below. The model consists of an input layer, two hidden layers and an output layer for the final result. The input layer takes different patient-specific parameters which are related to the patient and fibroid anatomy, experienced symptoms, previous treatment history for uterine fibroids, imaging parameters from MR and US imaging, and fibroid-specific features. The model then calculates individual weights for each of these parameters in the hidden layers which are then forwarded to the output layer for treatment outcome prediction. By iterating this process over multiple patient cases, the model essentially learns the general patterns in the data and can be used to predict the treatment outcome in new patients.
In clinical practice the screening of the patients suitable for HIFU treatments is typically conducted by the radiologist and/or gynaecologist in the case of uterine fibroids. However, this evaluation is always subjective and the results might thus differ between different practitioners. Utilising a neural network model in the patient screening stage could potentially improve the consistency of the HIFU treatment outcomes as well as reduce the workload required for the patient selection. Therefore, the aim of the project is to make the neural network model available as a tool for radiologists and clinicians to assist the patient selection for the HIFU treatments in uterine fibroids.
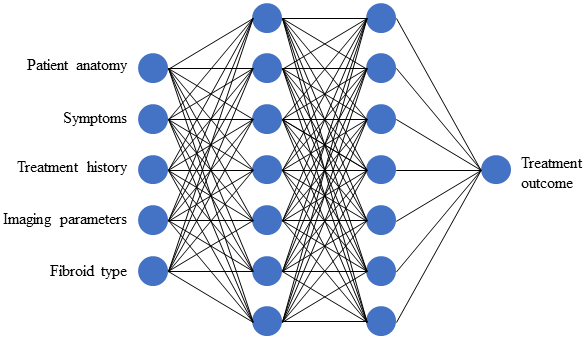